The World Health Organization’s 2021 World Malaria Report states that there were an estimated 241 million malaria cases and 627,000 deaths in 2020 in 85 malaria-endemic countries. To prevent malaria transmission, identifying and suppressing particular mosquito populations is critical.
These mosquitos breed in certain habitats, referred to as breeding sites. For malaria control groups, finding effective tools to identify and predict the location of breeding sites is paramount to defeat this life-threatening disease.
Earth Observation Data in Malaria Control Methods
Earth Observation data presents a major opportunity to identify these mosquito breeding sites. In the past, however, malaria control groups typically did not use it to identify and mitigate threatening mosquito populations. This was because Earth Observation data was historically more resource intensive, requiring field-based teams to validate the data and expensive tools to analyze the data.
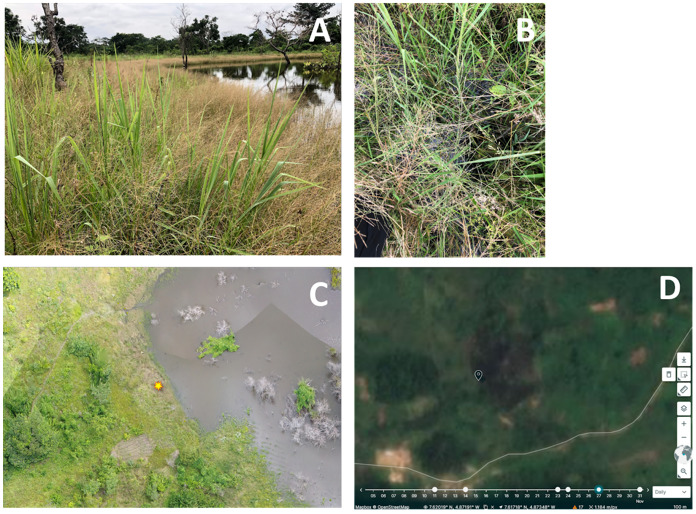
Using Deep Learning Approaches to Identify Breeding Sites
Luckily, Earth Observation data has become much more accessible in recent years. However, this data alone is not sufficient to identify breeding sites at scale as manually identifying all breeding sites on the planet would take inordinate effort. Researchers therefore also need tools to analyze the data to identify, and later train deep learning models to predict the location of breeding sites automatically at scale. Acquiring these tools could make it feasible for researchers to consider using imagery to analyze complex and developing environments. Researchers from the London School of Hygiene and Tropical Medicine and the Universidad Peruana Cayetano Heredia sought out to develop a repeatable workflow to characterize breeding sites using deep learning techniques with drone imagery in central Côte d’Ivoire to address the critical gaps that remain regarding data collection and analysis in malaria control. It was just a matter of finding a tool that was collaborative, accessible, and met the requirements for their research.

Labeling Aerial Imagery
Mosquitoes typically breed in small puddles with little vegetation. The first step in labeling imagery to build a machine learning model is therefore to label both the vegetated and non-vegetated water bodies where mosquitoes propagate. Once you have enough of these areas labeled you can then build a deep learning model that can identify such breeding grounds.
The Need for a User-Friendly, Collaborative Labeling Tool
In the case of the London School of Hygiene’s team, they needed a labeling tool that was collaborative to improve their workflow. Labeling imagery for training datasets is often tedious, therefore finding a user-friendly tool is important to accelerate progress. Collaborative capabilities would allow experts on the respective environments could approve the labeling after polygons were drawn. GroundWork met these requirements. It allowed the team to build a catalogue of training data for the project on an easy to use, collaborative platform that gave different uses different levels of drawing vs. approval permissions.
A More Effective Way to Suppress Malaria
The London School of Hygiene’s team sampled 189 potential mosquito breeding habitats of which 119 were identified as breeding sites. After labeling the imagery in GroundWork, they developed a deep learning approach to identify breeding grounds. They now have a repeatable workflow to control the source of malaria using Earth Observation data that they will use in their ongoing research. These findings will address the gaps that remain in data collection and analysis methods used to detect and suppress sources of malaria. Developing sophisticated deep learning methods for malaria control will make the process more efficient and effective. Azavea is proud to provide GroundWork, a data labeling tool that has made it possible for another resource-strained group to easily replicate the workflow outlined in this study. In this research, the group at the London School of Hygiene and Tropical Medicine and the Universidad Peruana Cayetano Heredia proved that a representative, thorough study is feasible with a combination of freely available Earth Observation data and tools.